It is important that the associations between both the genetic instrumental variable (IV) and exposure and the genetic IV and outcome in two-sample MR are from the same underlying population. Alternatively, at a minimum, there is evidence that the association between the IV and exposure is similar in the population used for the second sample (i.e., the source of association of the genetic IV and outcome).
Many genome-wide association studies (GWASs) that are used to identify genetic IVs associated with the exposure for an MR analysis are conducted in women and men combined. If these findings are then combined with data used to source information about the association between those same genetic IVs and the outcome in women only (for example, if the outcome of interest was breast or ovarian cancer), the assumption is that the association between the genetic IV and exposure does not differ between women and men. Ideally, the two samples would be from the same underlying population. If this is not possible (e.g., because aggregate data being used and the populations differ in the two samples), some attempt should be made to find evidence that the associations between the genetic IV and exposure is similar in the population used to source the association between the genetic IV and outcome, as in the original GWAS.
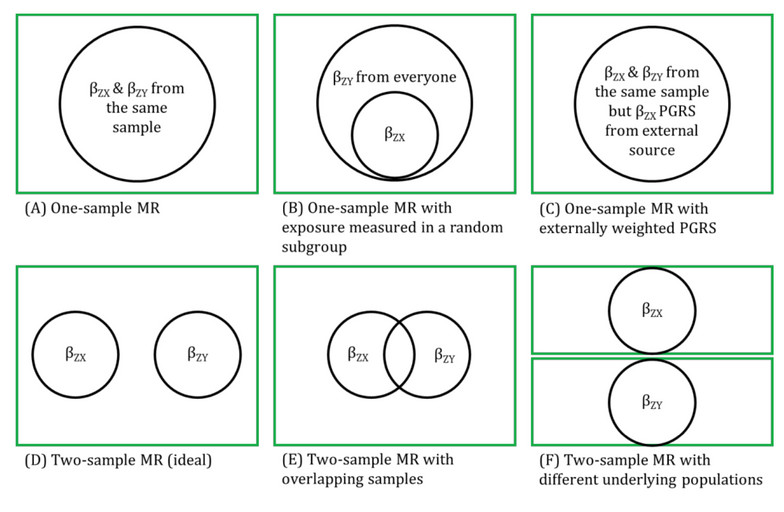
References
- Lawlor DA. Two-sample Mendelian randomization: opportunities and challenges. International Journal of Epidemiology 2016; 45: 908-915.
- Hartwig FP, Davies NM, Hemani G, Davey Smith G. Two-sample Mendelian randomization: avoiding the downsides of a powerful, widely applicable but potentially fallible technique. Int J Epidemiol 2016; 45: 1717-1726.
Other terms in 'Sources of bias and limitations in MR':
- Assortative mating
- Canalization
- Collider
- Collider bias
- Conditional F-statistic for multiple exposures
- Confounding
- Exclusion restriction assumption
- F-statistic
- Harmonization (in two-sample MR)
- Homogeneity Assumption
- Horizontal Pleiotropy
- Independence assumption
- INstrument Strength Independent of Direct Effect (InSIDE) assumption
- Intergenerational (or dynastic) effects
- Monotonicity assumption
- MR for testing critical or sensitive periods
- MR for testing developmental origins
- No effect modification assumption
- NO Measurement Error (NOME) assumption
- Non-linear MR
- Non-overlapping samples (in two-sample MR)
- Overfitting
- Pleiotropy
- Population stratification
- R-squared
- Regression dilution bias (attenuation by errors)
- Relevance assumption
- Reverse causality
- Statistical power and efficiency
- Vertical pleiotropy
- Weak instrument bias
- Winner's curse