MR analyses in which the associations between the genetic instrumental variable (IV) and exposure and the genetic IV and outcome are generated from different (non-overlapping) samples and summary-level data are used to derive the MR estimate.
Genetic IVs must fulfil the same MR assumptions. Genetic IVs associated with the exposure of interest are usually identified and summary-level data (e.g., betas, standard errors and p-values) describing the IV-exposure association are obtained from a genome-wide association study (GWAS) of the exposure. Summary-level data of those same exposure-related IVs are usually then extracted from a GWAS of the outcome. These summary-level data describing the IV-exposure and IV-outcome associations are then used to estimate the causal effect between the exposure and outcome. Equally, individual-level data can be used to derive these summary statistics if individual-level or individual participant data (IPD) is available. In this setting, there is more potential (than with summary-level data) for assessing the associations between the genetic IV with observed confounders of the exposure-outcome association and exploring interactions, non-linear or subgroup effects. With summary-level data, it may be difficult to ensure the two samples are from the same underlying population and non-overlapping. Two-sample MR is less prone to data overfitting than one-sample MR. In two-sample MR, weak instrument bias will tend to bias the estimate towards the null. Conversely, weak instrument bias in a one-sample MR will tend to bias the estimate towards the confounded multivariable regression estimate. Though, if there is overlap of participants in the two samples used for two-sample MR, the direction of any weak instrument bias in a two-sample setting will tend towards the confounded multivariable regression estimate (i.e., like in a one-sample setting with individual-level data) approximately proportional to the amount of overlap. Correct harmonization of alleles across the two samples is essential. The two samples should be from the same underlying population but should be (largely) non-overlapping. The IV-exposure and IV-outcome models must be correctly specified. NOTE: there are grey areas between one-sample and two-sample MR (e.g., in two-sample MR, where two samples have some overlap, as shown in Figure 2.6, or when one sample of individual-level data has been used to generate summary statistics that contribute to the derivation of the MR estimate using methods derived for a two-sample MR setting). In fact, some MR studies use a mixture of analyses conducted in both individual- and summary-level data, sometimes using the same population sample. Therefore, the way in which these methods are described has evolved in the literature to focus on the data source being used in MR analyses rather than the number of samples - i.e., "two-sample MR" is equivalent to "summary-level data MR" or "MR with summary-level data".
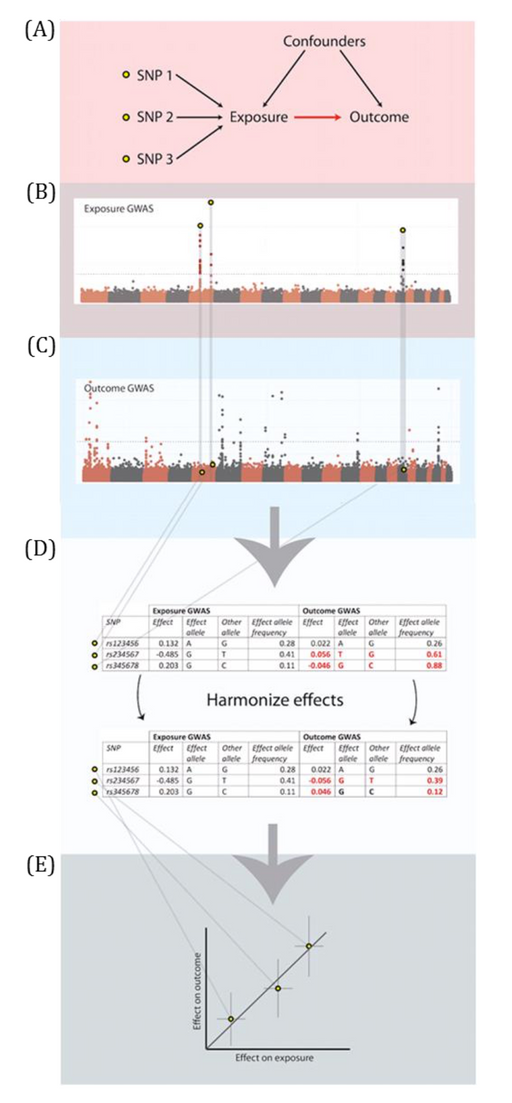
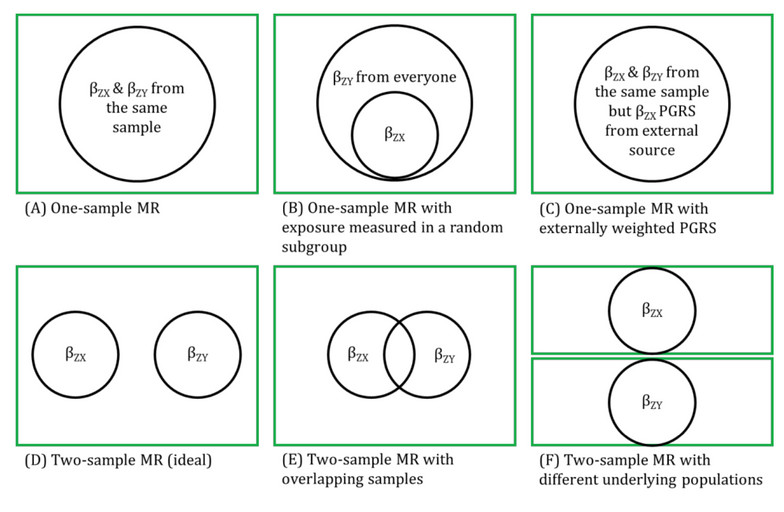
References
- Hemani G, Zheng J, Elsworth B, et al. The MR-Base platform supports systematic causal inference across the human phenome. Elife 2018; 7.
- Zhao Q, Wang J, Bowden J, Small DS. Two-Sample Instrumental Variable Analyses using Heterogeneous Samples. arxivorg/pdf/170900081 2018.
- Davey Smith G, Hemani G. Mendelian randomization: genetic anchors for causal inference in epidemiological studies. Hum Mol Genet 2014; 23: R89-R98.
- Lawlor DA. Two-sample Mendelian randomization: opportunities and challenges. International Journal of Epidemiology 2016; 45: 908-915.
Other terms in 'Definition of MR and study designs':
- Bidirectional MR
- Factorial MR
- Instrumental variable (IV)
- Mendelian randomization (MR)
- MR for drug targets
- MR with binary exposures
- Multivariable MR
- One-sample MR or MR with individual-level data
- Two-step or Mediation MR