The strengths of two-sample MR in terms of weak instrument bias being towards the null and minimising overfitting of the data assume that the two samples are completely independent of each other (i.e., there is no overlap of participants between the two samples).
In practice, when summary-level data from publicly available data are used, it may not be possible to determine whether there is overlap between samples. This is because many genome-wide association studies (GWASs) are conducted on consortia of many studies and, increasingly, GWASs are beginning to consistently utilized large-scale biobanks (e.g., UK Biobank). Thus, there is potential for overlap between some of the studies (and participants) that contribute to these consortia (such as the GIANT consortia GWAS for body mass index, waist-hip ratio and height, and the DIAGRAM consortia GWAS for diabetes). The likelihood that any weak instrument bias within a two-sample setting will resemble the one-sample setting, whereby the MR estimates will be biased towards the (possibly confounded) observational estimate, is approximately proportional to the amount of overlap. Therefore, if the genetic instrumental variables (IVs) are strong, participant overlap is less of a concern. Careful reading of consortia websites and supplementary material should be undertaken to determine which studies contribute to each of the samples used to characterise the associations between the IV-exposure and IV-outcome and, if possible, sensitivity analyses undertaken with overlapping studies removed. Additionally, methods such as bivariate linkage disequilibrium (LD) score regression can be used to estimate the level of overlap between two data sources.
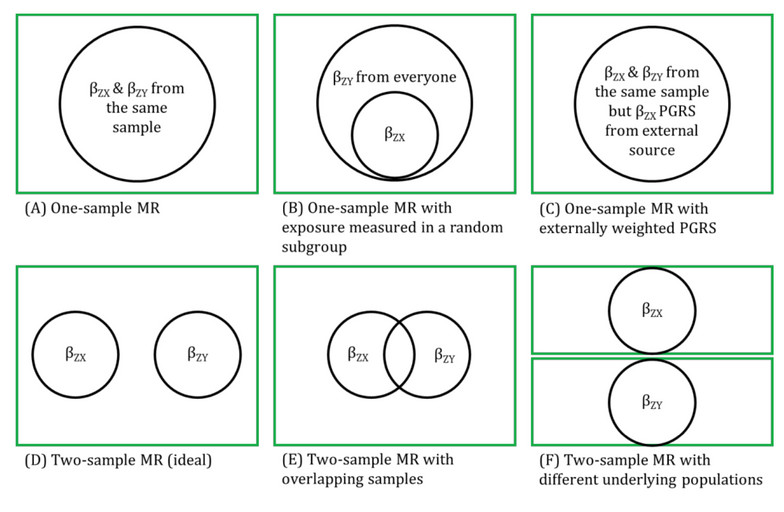
References
- Lawlor DA. Two-sample Mendelian randomization: opportunities and challenges. International Journal of Epidemiology 2016; 45: 908-915.
- Hartwig FP, Davies NM, Hemani G, Davey Smith G. Two-sample Mendelian randomization: avoiding the downsides of a powerful, widely applicable but potentially fallible technique. Int J Epidemiol 2016; 45: 1717-1726.
Other terms in 'Sources of bias and limitations in MR':
- Assortative mating
- Canalization
- Collider
- Collider bias
- Conditional F-statistic for multiple exposures
- Confounding
- Exclusion restriction assumption
- F-statistic
- Harmonization (in two-sample MR)
- Homogeneity Assumption
- Horizontal Pleiotropy
- Independence assumption
- INstrument Strength Independent of Direct Effect (InSIDE) assumption
- Intergenerational (or dynastic) effects
- Monotonicity assumption
- MR for testing critical or sensitive periods
- MR for testing developmental origins
- No effect modification assumption
- NO Measurement Error (NOME) assumption
- Non-linear MR
- Overfitting
- Pleiotropy
- Population stratification
- R-squared
- Regression dilution bias (attenuation by errors)
- Relevance assumption
- Reverse causality
- Same underlying population (in two-sample MR)
- Statistical power and efficiency
- Vertical pleiotropy
- Weak instrument bias
- Winner's curse