Traditionally, reverse causation is the phenomenon where an association in the direction of a hypothesised causal relationship between an exposure and outcome is observed but is due (at least in part) to (potentially undiagnosed or precursors of) prevalent "outcome" influencing the "exposure". In other words, what is considered the "outcome" is actually driving variation in the "exposure" and not in the hypothesised direction of causation from the exposure to the outcome.
For MR, in the presence of valid genetic instrumental variables (IVs) for both the "exposure" and "outcome", the presence of reverse causality can be tested in bidirectional MR. The phenomenon where the mechanism by which a genetic variant influences the "exposure" is actually via the "outcome" in an MR analysis is also usually termed reverse causation. For example, consider a genome-wide association study [GWAS of trait A (which will be considered the exposure in an MR analysis) that identifies a genetic variant associated with trait A. However, the biological mechanism by which that genetic variant influences trait A is via trait B. If trait B is then considered the outcome in that MR analysis, using the genetic variant that is associated with trait A (exposure) via trait B (outcome), can lead to biased MR estimates and spurious associations. In this context, the Instrument Strength Independent of Direct Effect (InSIDE) assumption in two-sample MR would be likely invalid and pleiotropy-robust methods may not be able to distinguish such phenomena from horizontal pleiotropy. To avoid these instances, greater knowledge of how the genetic variants influence our exposures and outcomes is required but this may only be possible with a small number of associated genetic variants. Visualising and comparing the strength of the associations between genetic variants and both the exposure and outcome can help assess these properties. Additionally, bidirectional MR and Steiger filtering can also be used to assess the relationships between the genetic variant and both the exposure and outcome.
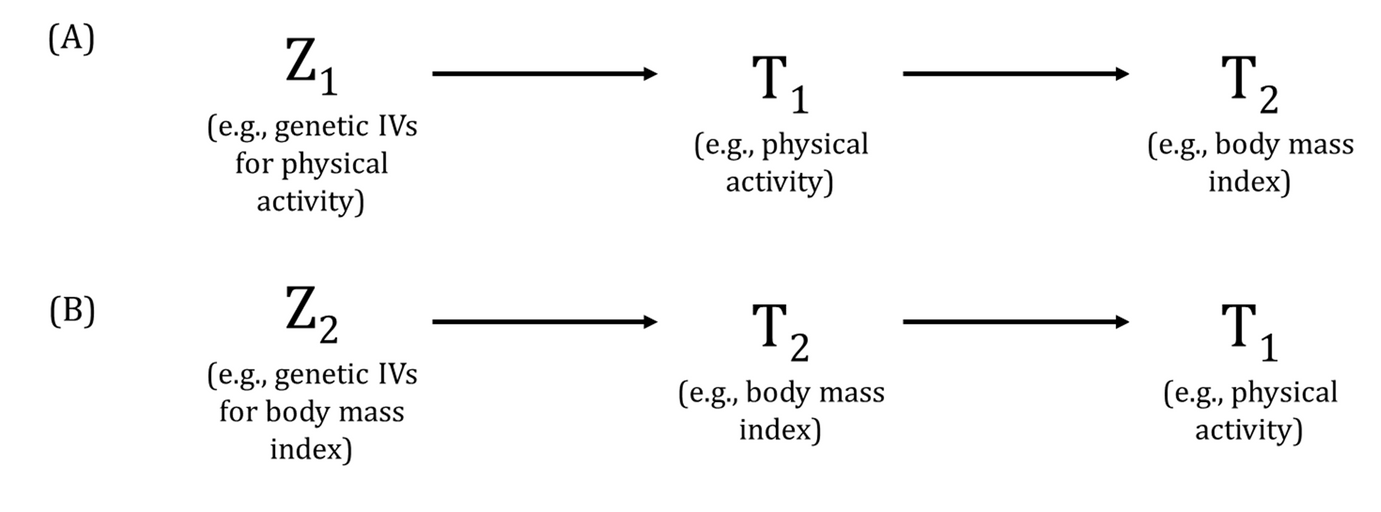
References
- Lawlor DA, Harbord RM, Sterne JAC, Timpson NJ, Davey Smith G. Mendelian randomization: using genes as instruments for making causal inferences in epidemiology. Statistic in Medicine 2008; 27: 1133-1163.
- Davey Smith G, Ebrahim S. "Mendelian randomisation": can genetic epidemiology contribute to understanding environmental determinants of disease? International Journal of Epidemiology 2003; 32: 1-22.
Other terms in 'Sources of bias and limitations in MR':
- Assortative mating
- Canalization
- Collider
- Collider bias
- Conditional F-statistic for multiple exposures
- Confounding
- Exclusion restriction assumption
- F-statistic
- Harmonization (in two-sample MR)
- Homogeneity Assumption
- Horizontal Pleiotropy
- Independence assumption
- INstrument Strength Independent of Direct Effect (InSIDE) assumption
- Intergenerational (or dynastic) effects
- Monotonicity assumption
- MR for testing critical or sensitive periods
- MR for testing developmental origins
- No effect modification assumption
- NO Measurement Error (NOME) assumption
- Non-linear MR
- Non-overlapping samples (in two-sample MR)
- Overfitting
- Pleiotropy
- Population stratification
- R-squared
- Regression dilution bias (attenuation by errors)
- Relevance assumption
- Same underlying population (in two-sample MR)
- Statistical power and efficiency
- Vertical pleiotropy
- Weak instrument bias
- Winner's curse